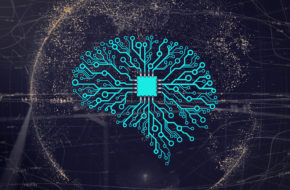
Technology has revolutionized how government, businesses, and nonprofits work and manage their data. Machine learning, a subset of artificial intelligence, utilizes algorithms to simplify work by pinpointing patterns in data to produce results. This process is rooted in the large amount of data that the model teaches the system to identify statistical patterns. An important distinction with machine learning is that humans do not write the code that tells the system how to differentiate a cat from a dog. The machine learning algorithm’s success is dependent on the quality of data it receives. The primary goal is for the computers to learn automatically without the need for human intervention, and the machine can adjust accordingly. Over the years, machine learning has been able to advance and be used to assist companies with data.
However, machine learning has some challenges; some being the limits of applying ethics, data, and interpretability. One of the biggest challenges of ethics is determining responsibility and trusting data and algorithms more than one’s judgment. A common example of ethics with machine learning is if a self-driving car hurts someone, whose fault is it? Transferring someone’s job to a computer raises ethical questions and discussions. Whereas the amount and quality of data greatly affect the model’s results. If there is not enough data nor accurate data, the algorithm will perform inadequately. Another prominent challenge for machine learning is interpretability. If professionals cannot understand the models from the algorithms, it is a problem for them to agree and trust the results and possible recommendations. There is still a need for humans to be involved in the machine learning process to ensure accurate and ethical performance.
An example of machine learning in recent news is the Library of Congress announced that they are looking at new ways to integrate humans in the process of expanding its digital library and developing tools to manage its collection. The reasoning behind having human-in-the-loop workflows is to “ensure properly tagging and verifying the metadata attached to the content.” While machine learning tools are utilized, the Library wants humans to be involved in the process to check the work to ensure that it is done correctly and ethically.
Praescient Analytics values and understands the importance of the human component when conducting data and intelligence analysis. While there has been a big push to use machine learning in many industries, humans still need to help fill the gaps and overcome the challenges as machine learning progresses and develops.